Well-formed formula: Difference between revisions
No edit summary |
|||
Line 1: | Line 1: | ||
{{redirect|Ancova|moth genus|Ancova (moth)}} | |||
[[Covariance]] is a measure of how much two variables change together and how strong the relationship is between them.<ref name="Howell">Howell, D. C. (2009) ''Statistical methods for psychology'' (7th ed.). Belmont: Cengage Wadsworth.</ref> '''Analysis of covariance (ANCOVA)''' is a [[general linear model]] which blends [[ANOVA]] and [[regression analysis|regression]]. ANCOVA evaluates whether population means of a [[dependent variable]] (DV) are equal across levels of a categorical [[independent variable]] (IV), while statistically controlling for the effects of other continuous variables that are not of primary interest, known as [[covariate]]s (CV). Therefore, when performing ANCOVA, the DV means are adjusted to what they would be if all groups were equal on the CV.<ref>Keppel, G. (1991). ''Design and analysis: A researcher's handbook'' (3rd ed.). Englewood Cliffs: Prentice-Hall, Inc.</ref> | |||
==Uses of ANCOVA== | |||
===Increase Power=== | |||
ANCOVA can be used to increase [[statistical power]]<ref>Tabachnick, B. G., & Fidell, L. S. (2007). ''Using Multivariate Statistics'' (5th ed.). Boston: Pearson Education, Inc.</ref> (the ability to find a [[Statistical significance|significant difference]] between groups when one exists) by reducing the within-group error [[variance]]. In order to understand this, it is necessary to understand the test used to evaluate differences between groups, the [[F-test]]. The ''F''-test is computed by dividing the explained variance between groups (e.g., gender difference) by the unexplained variance within the groups. Thus, | |||
<big>''F''</big> = <math>\frac{\mathit{MSbetween}}{\mathit{MSwithin}}</math> | |||
If this value is larger than a critical value, we conclude that there is a significant difference between groups. Unexplained variance includes error variance (e.g., individual differences), as well as the influence of other factors. Therefore, the influence of CVs is grouped in the denominator. When we control for the effect of CVs on the DV, we remove it from the denominator making ''F'' larger, thereby increasing your power to find a significant effect if one exists. | |||
[[File:ANCOVA - Partitioning Variance.jpg|left|Partitioning variance]] | |||
===Adjusting Preexisting Differences=== | |||
Another use of ANCOVA is to adjust for preexisting differences in nonequivalent (intact) groups. This controversial application aims at correcting for initial group differences (prior to group assignment) that exists on DV among several intact groups. In this situation, participants cannot be made equal through random assignment, so CVs are used to adjust scores and make participants more similar than without the CV. However, even with the use of covariates, there are no statistical techniques that can equate unequal groups. Furthermore, the CV may be so intimately related to the IV that removing the variance on the DV associated with the CV would remove considerable variance on the DV, rendering the results meaningless.<ref>Miller, G. A., & Chapman, J. P. (2001). Misunderstanding Analysis of Covariance. ''Journal of Abnormal Psychology, 110 (1),'' 40-48.</ref> | |||
==Assumptions of ANCOVA== | |||
There are five assumptions that underlie the use of ANCOVA and affect interpretation of the results:<ref name="Kutner ''et.al.''">Kutner, M. H., Nachtsheim, C. J., Neter, J., & Li, W. (2005). ''Applied Linear Statistical Models'' (5th ed.). New York, NY: McGraw-Hill/Irwin.</ref> | |||
===''Assumption 1: Normality of Residuals''=== | |||
The [[Errors_and_residuals_in_statistics|residuals (error terms)]] should be normally distributed.<br /> | |||
===''Assumption 2: Homogeneity of Variances''=== | |||
The error variances should be equal for different treatment classes.<br /> | |||
===''Assumption 3: Homogeneity of Regression Slopes''=== | |||
The slopes of the different regression lines should be equivalent, i.e., regression lines should be parallel among groups. <br /> | |||
===''Assumption 4: Linearity of Regression ''=== | |||
The regression relationship between the dependent variable and concomitant variables must be linear. <br /> | |||
===''Assumption 5: Independence of Error terms ''=== | |||
The error terms should be uncorrelated. <br /> | |||
The third issue, concerning the homogeneity of different treatment regression slopes is particularly important in evaluating the appropriateness of ANCOVA model. Also note that we only need the error terms to be normally distributed. In fact both the independent variable and the concomitant variables will not be normally distributed in most cases. | |||
==Conducting an ANCOVA== | |||
===''Test [[Multicollinearity]]''=== | |||
If a CV is highly related to another CV (at a correlation of .5 or more), then it will not adjust the DV over and above the other CV. One or the other should be removed since they are statistically redundant.<br /> | |||
===''Test the Homogeneity of Variance Assumption''=== | |||
Tested by [[Levene's test]] of equality of error variances. | |||
This is most important after adjustments have been made, but if you have it before adjustment you are likely to have it afterwards. <br /> | |||
===''Test the Homogeneity of Regression Slopes Assumption''=== | |||
To see if the CV significantly interacts with the IV, run an ANCOVA model including both the IV and the CVxIV interaction term. | |||
If the CVxIV interaction is significant, ANCOVA should not be performed. Instead, Green & Salkind<ref name="Green">Green, S. B., & Salkind, N. J. (2011). ''Using SPSS for Windows and Macintosh: Analyzing and Understanding Data'' (6th ed.). Upper Saddle River, NJ: Prentice Hall.</ref> suggest assessing group differences on the DV at particular levels of the CV. Also consider using a [[Moderation (statistics)|moderated regression analysis]], treating the CV and its interaction as another IV. Alternatively, one could use [[Mediation (statistics)|mediation analyses]] to determine if the CV accounts for the IV’s effect on the DV.<br /> | |||
===''Run ANCOVA Analysis''=== | |||
If the CVxIV interaction is not significant, rerun the ANCOVA without the CVxIV interaction term. | |||
In this analysis, you need to use the adjusted means and adjusted MSerror. The adjusted means (also referred to as least squares means, LS means, estimated marginal means, or EMM) refer to the group means after controlling for the influence of the CV on the DV.<br /> | |||
===''Follow-up Analyses''=== | |||
If there was a significant [[main effect]], it means that there is a significant difference between the levels of one IV, ignoring all other factors.<ref name="Howell"/> To find exactly which levels are significantly different from one another, one can use the same follow-up tests as for the ANOVA. | |||
If there are two or more IVs, there may be a [[Interaction (statistics)|significant interaction]], which means that the effect of one IV on the DV changes depending on the level of another factor. One can investigate the simple main effects using the same methods as in a [[Factor analysis|factorial ANOVA]]. | |||
==Power considerations== | |||
While the inclusion of a covariate into an ANOVA generally increases [[statistical power]] by accounting for some of the variance in the dependent variable and thus increasing the ratio of variance explained by the independent variables, adding a covariate into ANOVA also reduces the [[Degrees of freedom (statistics)|degrees of freedom]]. Accordingly, adding a covariate which accounts for very little variance in the dependent variable might actually reduce power. | |||
==See also== | |||
*[[MANCOVA]] (Multivariate analysis of covariance) | |||
==References== | |||
<references/> | |||
==External links== | |||
{{wikiversity}} | |||
* [http://www.southampton.ac.uk/~cpd/anovas/datasets/index.htm Examples of all ANOVA and ANCOVA models with up to three treatment factors, including randomized block, split plot, repeated measures, and Latin squares, and their analysis in R] | |||
* [http://faculty.vassar.edu/lowry/ch17pt1.html One-Way Analysis of Covariance for Independent Samples] | |||
* [http://journals.cambridge.org/action/displayAbstract?fromPage=online&aid=1296348 Use of covariates in randomized controlled trials by G.J.P. Van Breukelen and K.R.A. Van Dijk (2007)] | |||
{{Statistics|correlation|state=collapsed}} | |||
{{Experimental design|state=collapsed}} | |||
{{Least Squares and Regression Analysis|state=collapsed}} | |||
[[Category:Analysis of variance]] | |||
[[Category:Covariance and correlation]] |
Revision as of 00:54, 17 December 2013
Name: Jodi Junker
My age: 32
Country: Netherlands
Home town: Oudkarspel
Post code: 1724 Xg
Street: Waterlelie 22
my page - www.hostgator1centcoupon.info
Covariance is a measure of how much two variables change together and how strong the relationship is between them.[1] Analysis of covariance (ANCOVA) is a general linear model which blends ANOVA and regression. ANCOVA evaluates whether population means of a dependent variable (DV) are equal across levels of a categorical independent variable (IV), while statistically controlling for the effects of other continuous variables that are not of primary interest, known as covariates (CV). Therefore, when performing ANCOVA, the DV means are adjusted to what they would be if all groups were equal on the CV.[2]
Uses of ANCOVA
Increase Power
ANCOVA can be used to increase statistical power[3] (the ability to find a significant difference between groups when one exists) by reducing the within-group error variance. In order to understand this, it is necessary to understand the test used to evaluate differences between groups, the F-test. The F-test is computed by dividing the explained variance between groups (e.g., gender difference) by the unexplained variance within the groups. Thus,
F =
If this value is larger than a critical value, we conclude that there is a significant difference between groups. Unexplained variance includes error variance (e.g., individual differences), as well as the influence of other factors. Therefore, the influence of CVs is grouped in the denominator. When we control for the effect of CVs on the DV, we remove it from the denominator making F larger, thereby increasing your power to find a significant effect if one exists.
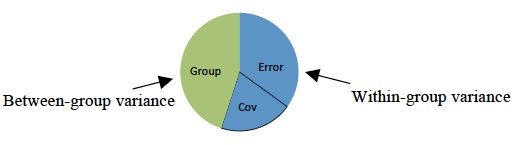
Adjusting Preexisting Differences
Another use of ANCOVA is to adjust for preexisting differences in nonequivalent (intact) groups. This controversial application aims at correcting for initial group differences (prior to group assignment) that exists on DV among several intact groups. In this situation, participants cannot be made equal through random assignment, so CVs are used to adjust scores and make participants more similar than without the CV. However, even with the use of covariates, there are no statistical techniques that can equate unequal groups. Furthermore, the CV may be so intimately related to the IV that removing the variance on the DV associated with the CV would remove considerable variance on the DV, rendering the results meaningless.[4]
Assumptions of ANCOVA
There are five assumptions that underlie the use of ANCOVA and affect interpretation of the results:[5]
Assumption 1: Normality of Residuals
The residuals (error terms) should be normally distributed.
Assumption 2: Homogeneity of Variances
The error variances should be equal for different treatment classes.
Assumption 3: Homogeneity of Regression Slopes
The slopes of the different regression lines should be equivalent, i.e., regression lines should be parallel among groups.
Assumption 4: Linearity of Regression
The regression relationship between the dependent variable and concomitant variables must be linear.
Assumption 5: Independence of Error terms
The error terms should be uncorrelated.
The third issue, concerning the homogeneity of different treatment regression slopes is particularly important in evaluating the appropriateness of ANCOVA model. Also note that we only need the error terms to be normally distributed. In fact both the independent variable and the concomitant variables will not be normally distributed in most cases.
Conducting an ANCOVA
Test Multicollinearity
If a CV is highly related to another CV (at a correlation of .5 or more), then it will not adjust the DV over and above the other CV. One or the other should be removed since they are statistically redundant.
Test the Homogeneity of Variance Assumption
Tested by Levene's test of equality of error variances.
This is most important after adjustments have been made, but if you have it before adjustment you are likely to have it afterwards.
Test the Homogeneity of Regression Slopes Assumption
To see if the CV significantly interacts with the IV, run an ANCOVA model including both the IV and the CVxIV interaction term.
If the CVxIV interaction is significant, ANCOVA should not be performed. Instead, Green & Salkind[6] suggest assessing group differences on the DV at particular levels of the CV. Also consider using a moderated regression analysis, treating the CV and its interaction as another IV. Alternatively, one could use mediation analyses to determine if the CV accounts for the IV’s effect on the DV.
Run ANCOVA Analysis
If the CVxIV interaction is not significant, rerun the ANCOVA without the CVxIV interaction term.
In this analysis, you need to use the adjusted means and adjusted MSerror. The adjusted means (also referred to as least squares means, LS means, estimated marginal means, or EMM) refer to the group means after controlling for the influence of the CV on the DV.
Follow-up Analyses
If there was a significant main effect, it means that there is a significant difference between the levels of one IV, ignoring all other factors.[1] To find exactly which levels are significantly different from one another, one can use the same follow-up tests as for the ANOVA. If there are two or more IVs, there may be a significant interaction, which means that the effect of one IV on the DV changes depending on the level of another factor. One can investigate the simple main effects using the same methods as in a factorial ANOVA.
Power considerations
While the inclusion of a covariate into an ANOVA generally increases statistical power by accounting for some of the variance in the dependent variable and thus increasing the ratio of variance explained by the independent variables, adding a covariate into ANOVA also reduces the degrees of freedom. Accordingly, adding a covariate which accounts for very little variance in the dependent variable might actually reduce power.
See also
- MANCOVA (Multivariate analysis of covariance)
References
- ↑ 1.0 1.1 Howell, D. C. (2009) Statistical methods for psychology (7th ed.). Belmont: Cengage Wadsworth.
- ↑ Keppel, G. (1991). Design and analysis: A researcher's handbook (3rd ed.). Englewood Cliffs: Prentice-Hall, Inc.
- ↑ Tabachnick, B. G., & Fidell, L. S. (2007). Using Multivariate Statistics (5th ed.). Boston: Pearson Education, Inc.
- ↑ Miller, G. A., & Chapman, J. P. (2001). Misunderstanding Analysis of Covariance. Journal of Abnormal Psychology, 110 (1), 40-48.
- ↑ Kutner, M. H., Nachtsheim, C. J., Neter, J., & Li, W. (2005). Applied Linear Statistical Models (5th ed.). New York, NY: McGraw-Hill/Irwin.
- ↑ Green, S. B., & Salkind, N. J. (2011). Using SPSS for Windows and Macintosh: Analyzing and Understanding Data (6th ed.). Upper Saddle River, NJ: Prentice Hall.
External links
- Examples of all ANOVA and ANCOVA models with up to three treatment factors, including randomized block, split plot, repeated measures, and Latin squares, and their analysis in R
- One-Way Analysis of Covariance for Independent Samples
- Use of covariates in randomized controlled trials by G.J.P. Van Breukelen and K.R.A. Van Dijk (2007)
Template:Statistics Template:Experimental design Template:Least Squares and Regression Analysis